Use Case – Aviation
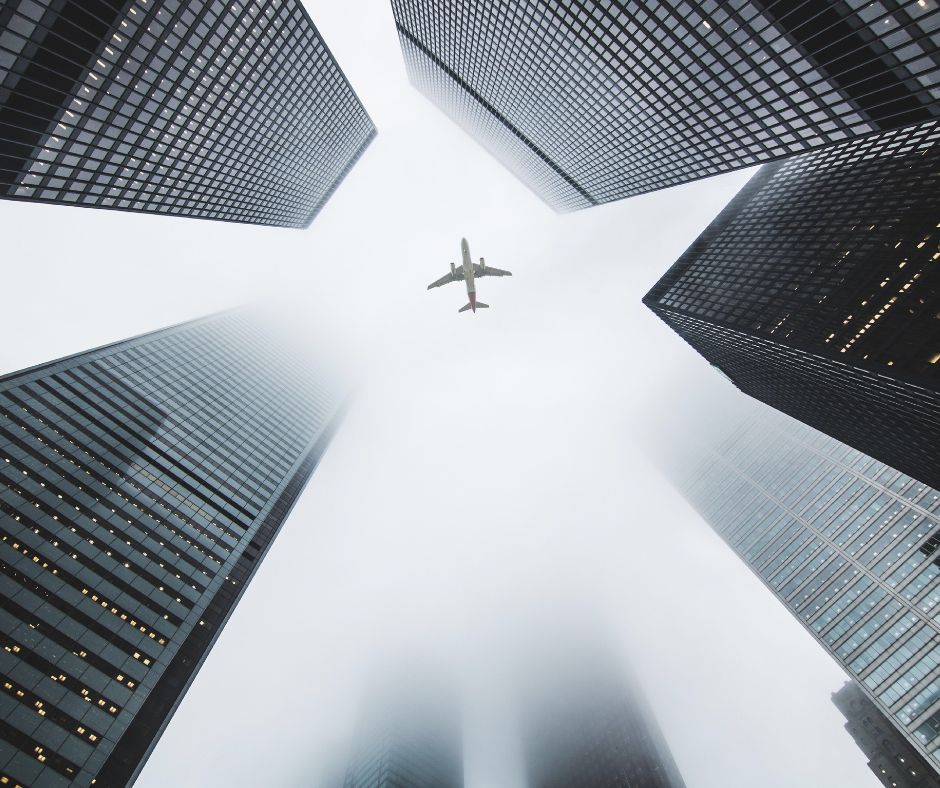
Aviation is facing major challenges: With emissions of 905 million tons of CO2 in 2018, it is responsible for 2.4% of global CO2 emissions (AV1). In addition, aviation traffic is expected to double by 2037 (AV2). Increasing emissions, increasing operational complexities: Experts expect a strong impact of quantum computing in dealing with these challenges, as today’s classical solutions reach their limits (AV3). This influence is expected in all fields of quantum computing, optimization, simulation, machine learning and cryptography, which is already being researched and tested by research institutions and companies:
From Flight Path Optimization, (LHIND: AV4), optimal assignment of flight activities in the context of arrival, parking and departure and passenger transfer (Flight Gate Assignment Problem, (AV5) to simulation of new materials as in the case of new bearing composites (BOEING: AV6) and fuel cells (AIRBUS: AV7). Optimization use cases in particular are characterized by the fact that the use of specific quantum computing hardware approaches, so-called annealers, can be expected to have an economic impact in aviation as early as the next 5-10 years (AV8).
Huge potential
In the case of flight path optimization, the economic potential of quantum computing is exemplified (AV9). Almost 50% of the operational costs are given by the direct flight operation costs. These include fuel consumption to a significant extent (AV10). Flight path optimization could thus reduce emissions of harmful greenhouse gases, as well as fuel and transport time. According to this share, flight optimization and associated relative savings of a few percent are an important economic factor and make flight path optimization a relevant problem.
Eliminate bottleneck
This is characterized primarily as a multivariable optimization problem subject to numerous constraints such as fuel consumption, flight time, aircraft weight, and air traffic control constraints. The large number of interrelated variables and the resulting high dimensionality pose a challenge for conventional optimization methods. Thus, classical methods have very limited ability to respond to the dynamically evolving environment and real-time constraints. This complexity requires the development of accurate models and algorithms that can adapt and respond in real-time. Current research shows initial promising results from quantum computers to overcome this bottleneck in the future (AV9).
Sources:
- AV1: Brandon Graver, “CO2 EMISSIONS FROM COMMERCIAL AVIATION”, 2018, URL: https://theicct.org/publication/co2-emissions-from-commercial-aviation-2018/, Aufruf: 27.09.23
- AV2: “ACI World study predicts global air traffic to double by 2037” 2019, URL: https://www.airport-technology.com/news/aci-world-study-global-air-traffic/, Aufruf: 27.09.23
- AV3: Imed Othmani et al., “Exploring quantum computing use cases for airlines“, 2020, URL: https://www.ibm.com/downloads/cas/XEANVAWQ, Aufruf: 27.09.23
- AV4: „Jury selects LHIND solution among the Top 5”, 2022, URL: https://www.lufthansa-industry-solutions.com/de-en/newsroom-downloads/news/quantum-climate-challenge-2022-team-of-experts-from-lufthansa-industry-solutions-among-the-top-5, Aufruf: 27.09.23
- AV5: Chai, Y., Funcke, L., Hartung, T., Jansen, K., Kuehn, S., Stornati, P., & Stollenwerk, T. (2023). Towards Finding an Optimal Flight Gate Assignment on a Digital Quantum Computer. http://arxiv.org/abs/2302.11595
- AV6: “Boeing seeks new ways to engineer strong, lightweight materials“, 2023, URL: https://www.ibm.com/case-studies/boeing, Aufruf: 27.09.23
- AV7: Bernd Reder, “BMW und Airbus nutzen Quantensystem für Forschung“ , 2023, URL: https://www.cio.de/a/bmw-und-airbus-nutzen-quantensystem-fuer-forschung,3716880, Aufruf: 27.09.23
- AV8: M., Seskir, Z. C., Posner, M. T., & Venegas-Gomez, A. (2023). Building a Quantum-ready Ecosystem. https://doi.org/10.1049/qtc2.12072
- AV9: Makhanov, H., Setia, K., Liu, J., Gomez-Gonzalez, V., & Jenaro-Rabadan, G. (2023). Quantum Computing Applications for Flight Trajectory Optimization. http://arxiv.org/abs/2304.14445
- AV10: Brian Beers, ”Which Major Expenses Affect Airline Companies?”, 2023, URL: https://www.investopedia.com/ask/answers/040715/what-are-major-expenses-affect-companies-airline-industry.asp#:~:text=2-,Cost%20of%20Fuel%20for%20Airlines,rise%2C%20this%20behavior%20is%20rewarded, Aufruf: 28.09.23